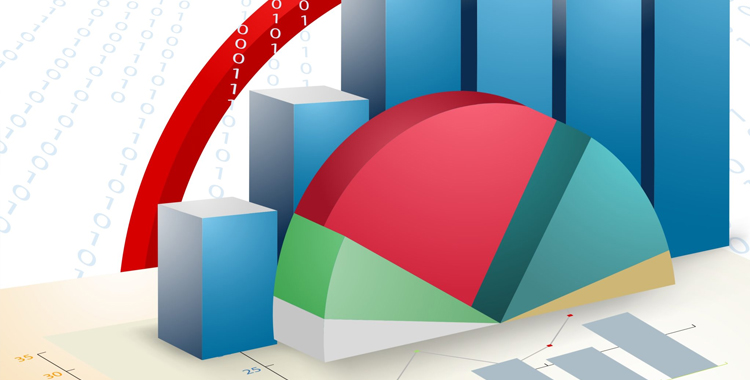
Community Data-Sharing 101: General Warehouse Design
It is easy to jump on the Collective Impact/Data-Driven Decision-Making bandwagon and just dive head-first into warehousing data. But this general, full-speed ahead approach is what has led to a situation I’ve seen in many communities all across the country: they’ve put a lot of time and effort into pulling a bunch of data together, but then it just sits there doing nothing.
In the Quad Cities, we didn’t even start the conversation about pooling data until we had a clear idea of what we wanted to pool the data for.
No two data warehouses are alike. Your data warehouse design will rely in large part on the partners you invite to contribute data to your project, what you hope to learn from the data you gather, and what you plan to do with what you’ve learned.
However, there are some general categories – functions your warehouse is designed to carry out – that are most common.
Don’t think of this list as a bunch of separate types of warehouse; think of it as a list of different possible functions your warehouse could serve. Most projects are a combination of several of these options at once:
- To track general trends in the overall population and identify community need
- To map and track the resources available in the community to meet that need
- To bring together indicators from different organizations (for example, neighboring school districts) who don’t measure their data in the same way
- To identify which groups (demographic, socio-economic, geographic) have the greatest need
- To track the progress of a specific group of individuals over time, to measure long-term impacts
- To track specific indicators (for example, the high school graduation rate) on a regular, and even “real time,” basis
- To break down data silos between organizations serving the same population
- To track the short- and long-term impacts of an intervention, or to compare one intervention’s impact to another’s
- To track the progress of a specific group of people for the purpose of case management.
For the Quad Cities, our biggest issue was the state border (between Iowa and Illinois, also known as the Mississippi River) that runs right across the middle of our community. We have 8 different school districts in 2 states, so we needed a single location where we could standardize all of the data. Also, a lot of our low-income families move a lot, and, in a bi-state community, that means they often move back and forth from one state to the other. By pooling data from the 8 districts in a single location, we’re able to continue to track these students so long as they remain in the region.
Because this was our focus, we were looking for software that could easily pool and standardize data from different sources and had an algorithm for matching individuals who appear in multiple databases. nFocus’ Community Solutions Data Warehouse software offered both of these functions.
Next, whatever purpose(s) you’ve picked for warehousing data will also dictate what level of data you’ll need. There are four general categories listed here from the easiest to hardest types of data to gain access to:
- Public data – Community-wide statistics, such as those available from the local health department or the U.S. Census Bureau. These data are useful in tracking broad trends (regional child obesity rates, for instance).
- Private data, aggregate – Statistics from a specific population (like all clients of a specific agency), reported as a group. You could find out, for example, what percentage of participants in a fitness program were able to lose weight, but not which participants lost weight and which didn’t.
- Private data, individual, de-identified – Data about individual members of a specific population, but with all identifying information (name, address, date of birth) removed. This data is useful to measure correlations between data points within the same data set (e.g., “What percentage of clients who started a personal savings account were also able to increase their credit rating by at least 50 points?”).
- Private data, individual, identified – You collect not just the outcomes data on each individual in the population, but their names and other identifying information as well. This level of data is most useful when trying to link data from two different sources (e.g., “How many students who earned 5 merit badges or more in Boy Scouts last year also had a 3.0 grade point average or higher at school?”)
The more personal the information you collect, the less freedom the organization has to share that data. They have a responsibility to protect the confidentiality of the clients – and often there are government regulations (FERPA for school district data, HIPAA for medical data) that come into play.
For example, if the sole purpose for our data warehouse in the Quad Cities was to pool and standardize data, then we would only need our student data at the “private, aggregate” level (and, in fact, for the first three years of the project, that’s what we were working with).
To be able to track students as they move and change districts, however, the data itself (such as a list of GPAs) was not enough. We needed to know the name and date of birth linked to each students’ GPA – we needed those data at the “private, individual, identified level.” That way, if a student moved halfway through the school year, we could match up the GPA that the two different districts reported and use the data warehouse to calculate an overall GPA for the student for the full school year.
When you’re dealing with higher level protected data, you need to be familiar with the legal issues involved, gain the trust of your data partners, and assure them that you’re going to set up the structures (not just the software and hardware you use for your Warehouse, but also the rules you create about how data should be stored and handled) to take care of their data as well as they would.
Individual-level, identified data is by far the most difficult to gain access to, but it is also the most versatile and exact. Data warehouse design is always a balance between what level of data (individual vs. aggregate; identified vs. de-identified) your question requires and what your partners are willing to – and/or are legally allowed to – share. Attempts to create a data warehouse to hold these high-level data sets will require months, and sometimes years, of work: building partnerships, gaining trust, and designing security protocols. If you succeed, however, you will have created a powerful tool which will better help you zero in on community need and the best approaches to addressing that need – a scalpel, rather than a sledgehammer.
Community Data-Sharing 101 Blog Series
- The Importance of the Question
- General Warehouse Design
- Partner Engagement
- Building Partnerships
- Picking a Data Steward Partner
- Demystifying the Data-Sharing Agreement
- The Data-Sharing Agreement as a Living Document
To learn more about the Quad Cities’ educational initiatives and how nFocus Solutions is impacting the community, read the case study, The Quad Cities: Making Shared Data a Reality.